The Role of Machine Learning in Renewable Energy Optimization
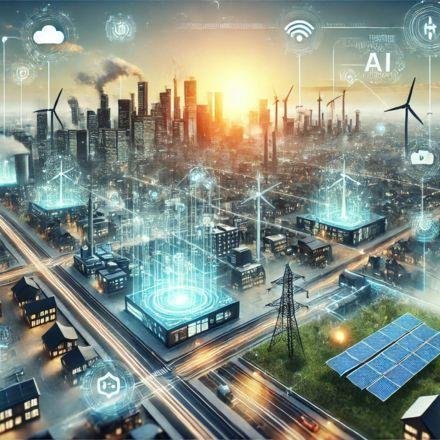
As the world shifts towards sustainable energy sources, the integration of advanced technologies is becoming crucial for maximizing efficiency and reliability. One of the most transformative technologies in this field is machine learning. With its ability to process vast amounts of data and generate predictive insights, machine learning in renewable energy is reshaping how energy is produced, stored, and distributed. By leveraging AI-driven analytics, companies can enhance energy efficiency with AI solution applications, leading to more reliable and sustainable energy management.
Predictive Maintenance for Renewable Energy Infrastructure
One of the most significant applications of machine learning in renewable energy is predictive maintenance. Wind turbines, solar panels, and hydroelectric systems require regular maintenance to operate at peak efficiency. Traditional maintenance methods often rely on scheduled inspections, which may lead to unnecessary costs or unexpected failures.
Machine learning algorithms analyze real-time performance data from sensors embedded in renewable energy equipment. By detecting anomalies and predicting failures before they occur, energy providers can reduce downtime, lower maintenance costs, and extend the lifespan of their assets. This proactive approach significantly enhances energy efficiency with AI solution applications.
Smart Grid Optimization
Renewable energy sources like wind and solar are inherently variable, making grid stability a critical challenge. Machine learning models help balance supply and demand by analyzing weather patterns, historical energy usage, and grid conditions in real time. AI-powered smart grids can automatically adjust energy distribution based on real-time data, ensuring a stable and efficient power supply.
By integrating machine learning in renewable energy, utilities can optimize grid operations, reduce energy wastage, and enhance the overall resilience of the power network. These intelligent systems can also help in forecasting power generation and demand, further improving energy management.
Enhanced Energy Storage Management
Energy storage is a key factor in the success of renewable energy adoption. Batteries store excess energy generated during peak production hours and release it when demand is high. However, inefficient storage management can lead to energy loss and a reduced battery lifespan.
Machine learning algorithms optimize energy storage by analyzing consumption patterns and battery performance. AI can determine the best times to charge and discharge batteries, preventing energy wastage and maximizing efficiency. This approach ensures that renewable energy sources are utilized effectively, reducing dependency on fossil fuels and lowering carbon footprints.
Optimizing Solar and Wind Energy Production
Solar and wind energy production depends on environmental factors such as sunlight intensity and wind speed. Machine learning models leverage historical and real-time data to predict energy generation accurately. By incorporating AI-driven weather forecasting and data analytics, renewable energy companies can enhance production efficiency and make informed decisions on energy distribution.
For example, solar farms use AI to adjust the angle of solar panels for maximum sunlight exposure, while wind farms optimize turbine blade positioning for optimal energy capture. This level of precision leads to improved energy efficiency with AI solution applications, ultimately increasing the viability of renewable energy projects.
Demand Forecasting and Energy Consumption Optimization
One of the biggest challenges in renewable energy management is balancing supply and demand. Traditional energy grids rely on estimations that may not always align with actual consumption patterns. Machine learning algorithms analyze real-time energy usage and predict future demand with high accuracy.
By leveraging AI-driven demand forecasting, energy providers can allocate resources efficiently, minimize energy wastage, and reduce reliance on backup fossil fuel power plants. This not only improves the overall efficiency of energy systems but also supports a more sustainable energy ecosystem.
AI-Powered Climate Impact Analysis
Climate change has a direct impact on renewable energy generation. Unpredictable weather patterns can affect the efficiency of solar, wind, and hydroelectric power. Machine learning models analyze climate trends and provide actionable insights to mitigate risks associated with fluctuating environmental conditions.
By understanding long-term climate changes, energy providers can develop resilient strategies for renewable energy production. AI-powered analytics enable proactive decision-making, ensuring that renewable energy sources remain a reliable and viable alternative to conventional power generation methods.
Conclusion
Machine learning in renewable energy is revolutionizing the way we produce, store, and distribute clean energy. By enhancing predictive maintenance, optimizing grid operations, improving energy storage management, and forecasting demand, AI-driven solutions are paving the way for a more efficient and sustainable energy future. The integration of energy efficiency with AI solution applications is not only reducing operational costs but also accelerating the transition to a greener planet. As technology advances, the role of machine learning in renewable energy will continue to expand, making renewable power more reliable, cost-effective, and accessible on a global scale.